         
Tutorial Sessions/Invited Talks
All tutorials and invited talks are free to registered
conference attendees of all conferences held at
WOLDCOMP'13. Those who are interested in attending one
or more of the tutorials are to sign up on site at the
conference registration desk in Las Vegas. A complete &
current list of WORLDCOMP Tutorials
can be found
here.
In addition to tutorials at other conferences,
DMIN'14 aims at providing a set of tutorials dedicated
to Data Mining topics. The 2007 key tutorial was given
by Prof. Eamonn Keogh on Time Series Clustering. The
2008 key tutorial was presented by Mikhail Golovnya
(Senior Scientist, Salford Systems, USA) on Advanced
Data Mining Methodologies. DMIN'09 provided four
tutorials presented by Prof. Nitesh V. Chawla on Data
Mining with Sensitivity to Rare Events and Class
Imbalance, Prof. Asim Roy on Autonomous Machine Learning,
Dan Steinberg (CEO of Salford Systems) on Advanced Data
Mining Methodologies, and Peter Geczy on Emerging
Human-Web Interaction Research. DMIN'10 hosted a
tutorial presented by Prof. Vladimir Cherkassky on
Advanced Methodologies for Learning with Sparse Data. He
was a keynote speaker as well (Predictive Data Modeling
and the Nature of Scientific Discovery). In 2011, Gary
M. Weiss (Fordham University, USA) presented a tutorial
on Smart Phone-Based Sensor Data Mining. Michael Mahoney
(Stanford University, USA) gave a tutorial on Geometric
Tools for Identifying Structure in Large Social and
Information Networks. DMIN'12 hosted a talk given by
Sofus A. Macskassy
(Univ. of Southern California, USA) on Mining
Social Media: The Importance of Combining Network and
Content as well as a talk given by Haym Hirsh (Rutgers
University, USA): Getting the Most Bang for Your Buck:
The Efficient Use of Crowdsourced Labor for Data
Annotation. Professor Hirsh was a WORLDCOMP keynote
speaker, too.
In addition, we hosted tutorials
and invited talks held by Peter Geczy on Web Mining,
Data Mining and Privacy: Water and Fire?,
and Data Mining in Organizations. DMIN'13 hosted the
following tutorials:
EXTENSIONS
and APPLICATIONS of UNIVERSUM LEARNING
presented by
Vladimir
Cherkassky (Dept. Electrical & Computer Eng.,
University of Minnesota,
Minneapolis, USA),
Visualization
& Data Mining for High Dimensional Datasets
presented by Alfred
Inselberg, (School of Mathematical Sciences, Tel
Aviv University, Tel Aviv, Israel)
as well as invited talks:
Big Data = Big Challenges?
given by
Peter Geczy (National Institute of Advanced Industrial
Science and Technology (AIST), Japan)
and
The Problem
of Induction: When Karl Popper meets Big Data
given by Vladimir Cherkassky.
DMIN'14 will be hosting the following
tutorials/invited talks:
Tutorials
Tutorial |
Speaker |
Gary M. Weiss,
Associate Professor & Director of Wireless
Sensor Data Mining (WISDM) Lab, Dep. of Computer
and Information Science, Fordham Univesity,
Bronx, NY, USA |
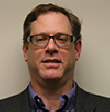 |
Topic/Title |
Smartphone Sensor
Mining Applications: Ubiquitous Possibilities
|
Date & Time |
July 23, 2014
(Wednesday) - 5:40pm |
Location |
Ballroom 1 |
Description |
Smart phones have
exploded in popularity over the past half dozen
years and these devices are now not just
ubiquitous, but powerful and packed with sensors
such as an accelerometer, gyroscope, GPS,
compass, barometer, audio sensor (microphone),
image sensor (camera), and light sensor. We are
now also beginning to see these sensors migrate
to smartwatches, which are primed to explode in
popularity. These mobile sensors make exciting
new applications possible. In this tutorial I
will survey some of these sensor mining
applications, discuss the underlying technology
and technology challenges, and describe the role
of data mining in these applications. The new
breed of applications that have recently hit the
market will allow our mobile devices to become
truly intelligent and context-aware, and allow
them to learn a lot about us and our immediate
environment. This tutorial is intended as an
introduction to the area and is appropriate for
anyone who is interested in the area. |
Short Bio |
Gary Weiss is a
faculty member in the department of Computer and
Information Science at Fordham University in New
York City. He is the Director of the Wireless
Sensor Data Mining (WISDM) Lab, which explores
how smartphone and other mobile sensors can be
used to support human activity recognition and
related applications. The WISDM Lab, which is
supported by grants from the U.S. National
Science Foundation, Google, and several other
corporations, recently released the actitracker
activity tracking app (actitracker.com). Prior
to coming to Fordham, Dr. Weiss worked at AT&T
Labs as a software engineer, expert system
developer, and finally as a data scientist. He
received a B.S. degree in
Computer
Science from
Cornell
University, an M.S. degree in
Computer
Science from
Stanford
University, and a Ph.D. degree in
Computer
Science from
Rutgers
University. He has published over fifty
papers in machine learning and data mining. |
Invited Talks
Invited Talk A |
Speaker |
Diego Galar,
Division of Operation and Maintenance
Engineering,
Luleå University of Technology, 971 87 Lulea,
Sweden |
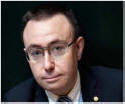 |
Topic/Title |
Big Data
Issues for Mining Knowledge in Maintenance
Information Systems |
Date & Time |
July 22, 2014 (Tuesday),
12:00-01:00pm |
Location |
Ballroom 1 |
Description |
ABSTRACT
Maintenance is a strategic process and/or
service all over the word. The effective
monitoring of the assets is a key task in order
to guarantee efficient and safe exploitation.
Current assets, with plenty of sensors' already
installed and pervasive computing on them
generate a huge of data along their day‐today
working as well as their maintenance. In this
scenario, asset managers host a large number of
diverse systems where data, regarding different
aspects of their activity, are stored. In most
cases, these data are captured, stored and
processed by different - often incompatible
systems -, and further managed by independent
departments and not shared at all.
In addition, it is
fairly frequent that while large amounts of data
are gathered only a small fraction of it is used
for a specific purpose; the remainder is simply
saved, or even worse, discarded. Important
information and knowledge are buried within
those extends of data. They could be discovered
if the data were properly organized and
processed. A number of unused techniques and
paradigms in Information Technology allow for
this knowledge discovery. On the one hand, Cloud
Computing brings a new service delivery paradigm
allows for pay-as-you-go services, which adapt
to the customer needs, without requiring
expensive data centers' infrastructures. From
the customer point of view, Cloud Computing
brings the possibility of requesting computing
resources, storage, and network bandwidth - as
needed. This paradigm can be used both from
public services or even within‐house private
services.
On the other hand,
Data Mining techniques, developed along fifteen
years have allowed the discovery of non‐trivial
knowledge from large databases. As the computing
resources increase in their power and decrease
in their prices, the capture and storage of data
are becoming increasingly affordable leading to
huge Big Data techniques to improve asset
monitoring and management stores of data. This
is increasing in several dimensions, not just
size but also, variety - structure,
semi-structured, non-structured -, speed of
gathering, ... to face their management and
processing new computing techniques are required.
They are all included under a new term: Big Data.
It refers to systems, algorithms, and procedures
suitable to process data sets, which largely
overcome the capacity of current single
computers. Big Data is one-term drawing
attention of many companies and institutions all
over the world. Most organizations are speeding
up their data processing strategies towards Big
Data. This means a clear recognition by industry,
agencies and public institutions. That is why,
the goal of this talk is to address the current
challenges of big data in maintenance; i.e. the
analysis, design and implementation of systems
that allow for the effective exploitation of
data for asset managers.
[see also Special
Session on Data Mining Applications for Advanced
Manufacturing [more]] |
Short Bio |
Prof. Diego Galar
has a Msc in Telecommunications and a PhD degree
in Manufacturing from the University of
Saragossa. He has become Professor in several
universities, including the University of
Saragossa or the European University of Madrid.
He also was a senior researcher in I3A,
Institute for engineering research in Aragon,
director of academic innovation and subsequently
pro-vice-chancellor of the university.
In industry, he
has been also technological director and CBM
manager. He has authored more than hundred
journal and conference papers, books and
technical reports in the field of maintenance.
Currently, he
is Professor of Condition Monitoring in the
Division of Operation and Maintenance in LTU,
Luleå University of Technology, where he is
coordinating several EU-FP7 projects related to
different maintenance aspects and is also
involved in the SKF UTC centre located in Lulea
focused in SMART bearings.
He is also
visiting Professor in the University of
Valencia, Polytechnic of Braganza (Portugal),
Valley University (Mexico), Sunderland
University (UK) and NIU (USA). |
Invited Talk B |
Speaker |
Peter Geczy
National Institute of Advanced Industrial
Science and Technology (AIST), Japan |
 |
Topic/Title |
Data Science
Emergence |
Date & Time |
July 23, 2014 (Wednesday),
12:00-01:00pm |
Location |
Ballroom 1 |
Description |
Explosion of
digital data and its diversity over the past
decade has been challenging scientists and
practitioners in a range of areas. Vast amounts
of data are being produced every day. It is
estimated that the growth rate is exponential
and no saturation point, or gradual flattening,
is expected to occur in a near-future. The
present trend indicates that the rapid data
expansion will interpenetrate into a broader
spectrum of scientific, commercial and social
spheres. The necessity to cope with these
challenges gave birth to Data Science - an
emerging field of scientific and educational
endeavor. Data Science is a novel
interdisciplinary domain that has been
progressively forming over the past decade. It
addresses two pressing needs: a coherent
scientific approach to rising data related
challenges and a growing demand for education of
new professionals - data scientists. We shall
explore both scientific and professional aspects
of these emerging initiatives. |
Short Bio |
Dr. Peter Geczy is
with the National Institute of Advanced
Industrial Science and Technology (AIST). He
also held positions at the Institute of Physical
and Chemical Research (RIKEN) and the Research
Center for Future Technologies. His
interdisciplinary scientific interests encompass
domains of data science, human interactions and
behavior, social intelligence technologies,
privacy, information systems, knowledge
management and engineering, artificial
intelligence, and adaptable systems. His recent
research focus also extends to the spheres of
service science, engineering, management, and
computing. He received several awards in
recognition of his accomplishments. Dr. Geczy
has been serving on various professional boards
and committees, and has been a distinguished
speaker in academia and industry. |
|
|